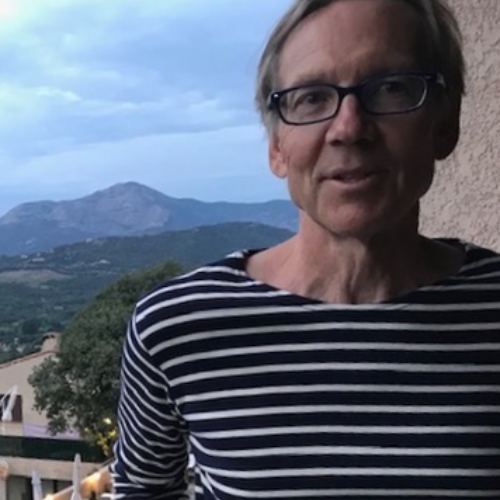
Member Since 1981
Paul A. Johnson
Senior Scientist and Fellow, Los Alamos National Laboratory
AGU Research
Search
Filters
Clear All
Seismic Features Predict Ground Motions During Repeating Caldera Collapse Sequence
GEOPHYSICAL RESEARCH LETTERS
10 june 2024
Christopher W. Johnson, Paul A. Johnson
Applying machine learning to continuous acoustic emissions, signals previously deemed noise, from laboratory faults and slowly slipping subduction&...
Seismic fingerprint predicts ground motions during the 2018 Kilauea collapse sequence
RUPTURE AND DEFORMATION PROCESSES, FROM SLOW TO FAST AND FROM MICRO TO MACRO I ORAL
mineral and rock physics | 14 december 2023
Christopher W. Johnson, Paul A. Johnson
Applying machine learning has demonstrated that acoustic emissions from laboratory earthquake faults contain signatures regarding the workings of the ...
View Abstract
Mapping Glacier Basal Sliding Applying Machine Learning
JOURNAL OF GEOPHYSICAL RESEARCH: EARTH SURFACE
08 november 2023
Josefine Umlauft, Christopher W. Johnson, Phillipe...
During the RESOLVE project (“High‐resolution imaging in subsurface geophysics: development of a multi‐instrument platform for int...
Machine Learning Developments and Applications in Solid‐Earth Geosciences: Fad or Future?
JOURNAL OF GEOPHYSICAL RESEARCH: SOLID EARTH
18 january 2023
Yunyue E. Li, Gregory C. Beroza, Andrew Curtis, Pa...
After decades of low but continuing activity, applications of machine learning (ML) in solid Earth geoscience have exploded in popularity. This spe...
Predicting Future Laboratory Fault Friction Through Deep Learning Transformer Models
GEOPHYSICAL RESEARCH LETTERS
10 october 2022
Kun Wang, Christopher W. Johnson, Kane Bennett, Pa...
Machine learning models using seismic emissions as input can predict instantaneous fault characteristics such as displacement and friction in labor...
Measuring the orientation of SHmax using ambient seismic noise
GEOPHYSICAL CONSTRAINTS ON CRUSTAL STRESS AND STRUCTURE IN THE AGE OF MACHINE LEARNING I ORAL
tectonophysics | 17 december 2021
Andrew A. Delorey, Goetz Bokelmann, Christopher W....
The orientation of SHmax, the maximum horizontal compressive stress, is important for geodynamical modeling and understanding earthquake hazards, as w...
View Abstract
Predicting the Future Fault Frictional Coefficient in a Shear Experiment Applying Deep Learning
FAULT-SLIP ACCELERATION: FROM SLOW TO FAST, FROM THE LAB TO THE FIELD, AND IN BETWEEN I ORAL
mineral and rock physics | 15 december 2021
Kun Wang, Christopher W. Johnson, Kane Bennett, Pa...
In a canonical paper (Rouet-LeDuc et al., 2017) it was shown that the acoustic emission (AE) recorded in laboratory shear experiments is rich with inf...
View Abstract
Transfer Learning From Numerical Experiments Predict Fault Friction in the Laboratory
DECODING GEOPHYSICAL SIGNATURES WITH MACHINE LEARNING: NOVEL METHODS AND RESULTS VI POSTER
seismology | 15 december 2021
Kun Wang, Christopher W. Johnson, Kane Bennett, Pa...
Data-driven machine-learning for predicting instantaneous and future fault-slip characteristics in laboratory experiments has recently progressed mark...
View Abstract