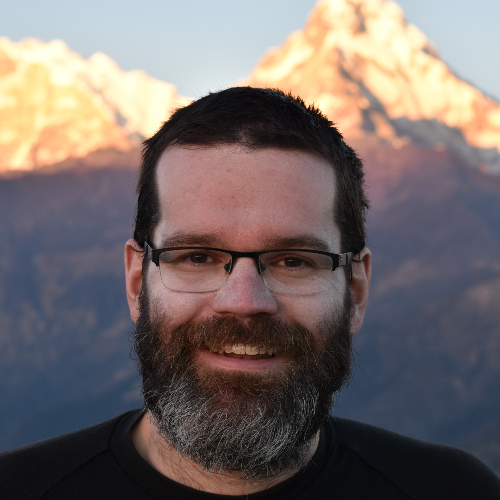
Colin Gleason is an outstanding hydrologist and one of the world’s leading researchers working today in the field of remote sensing for fluvial geomorphology. He is responsible for multiple groundbreaking findings at the interface of hydrology and geomorphology across many scales.
Despite only completing his Ph.D. in 2016, Dr. Gleason’s work has already contributed exciting new lines of research and deepened our understanding of a number of long-standing debates in fluvial geomorphology, in particular through his discovery of “at-many-stations hydraulic geometry,” or AMHG. Classical hydraulic geometry theory was originally formulated in the 1950s and was studied extensively in the 1960s, 1970s, and 1980s. Received wisdom was that there was little left to learn, yet as a Ph.D. student Dr. Gleason spotted a connection that had been missed. Specifically, what Colin uncovered was that the coefficients in hydraulic geometry theory were closely correlated in a log linear manner. In further work, Dr. Gleason has provided a theoretical explanation for the empirical AMHG result and shown that it is fully consistent with previous work. Not only is AMHG a major scientific breakthrough, it has also proved exceptionally powerful and has led to (1) new methods to estimate river discharge from space that are currently being implemented operationally in the forthcoming Surface Water and Ocean Topography (SWOT) satellite mission; (2) new insights into arctic hydrology; and (3) a fundamental reconsideration of the broad canon of hydraulic geometry theory.
Moreover, Dr. Gleason contributes substantially to his discipline through various important roles within the Mission Science Team for the recently launched NASA/CNES SWOT satellite where he coleads the Discharge Algorithm Working Group. This team is responsible for producing the operational architecture to estimate discharge along all global rivers greater than 50 meters wide (i.e., at millions of sites globally). This architecture needs to run in near-real time and ingest many terabytes of data every day to produce outputs that will be used (and critically evaluated) by many tens of thousands of scientists globally. Colin’s leadership has thus resulted in a critical component and backbone of the SWOT mission data system, and in guiding the development of this over the past decade, Colin has performed an immeasurable service for this community.
—Paul Bates, University of Bristol, Bristol, U.K.
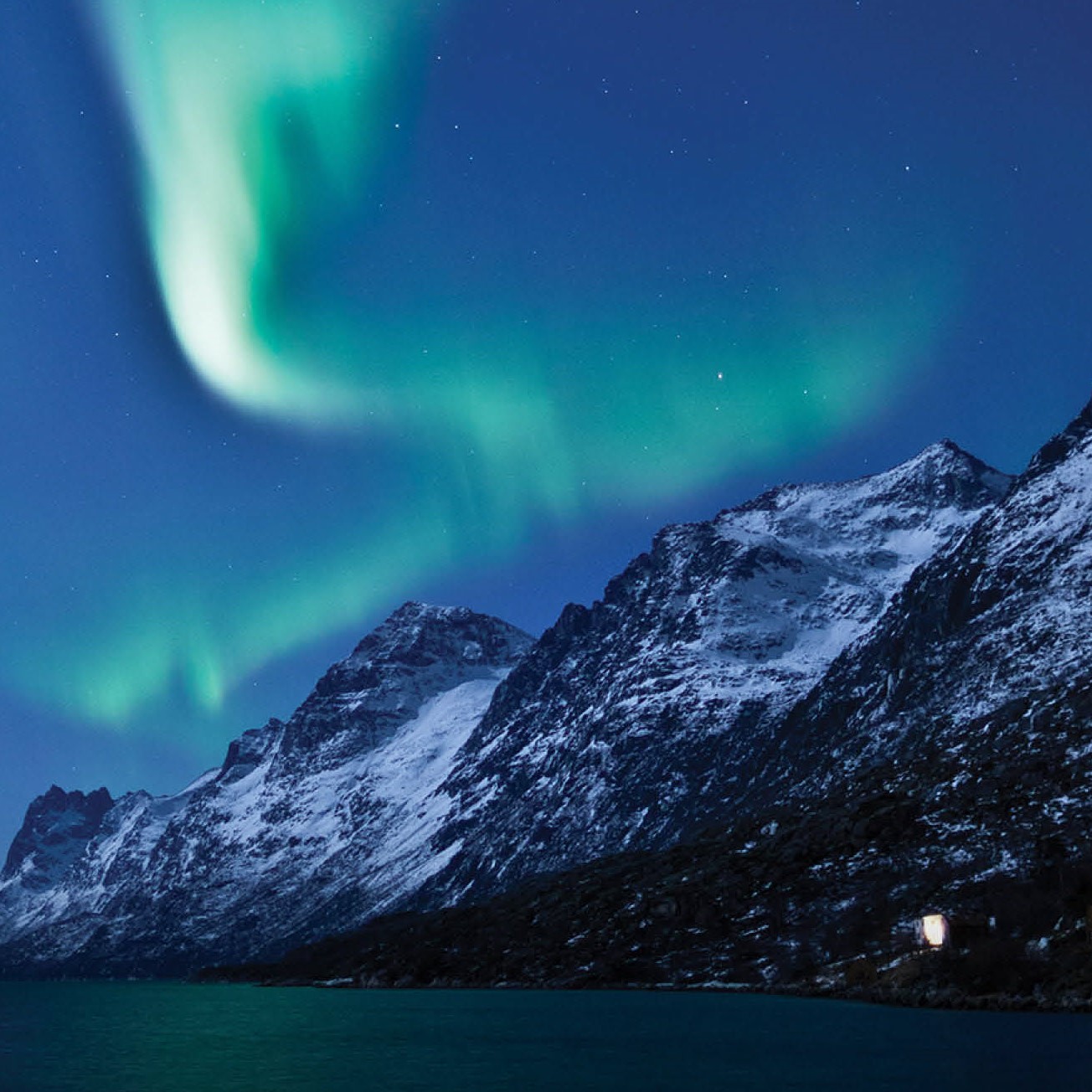
Inland waters emit significant amounts of carbon dioxide (CO2) to the atmosphere; however, the global magnitude and source distribution of inland w...
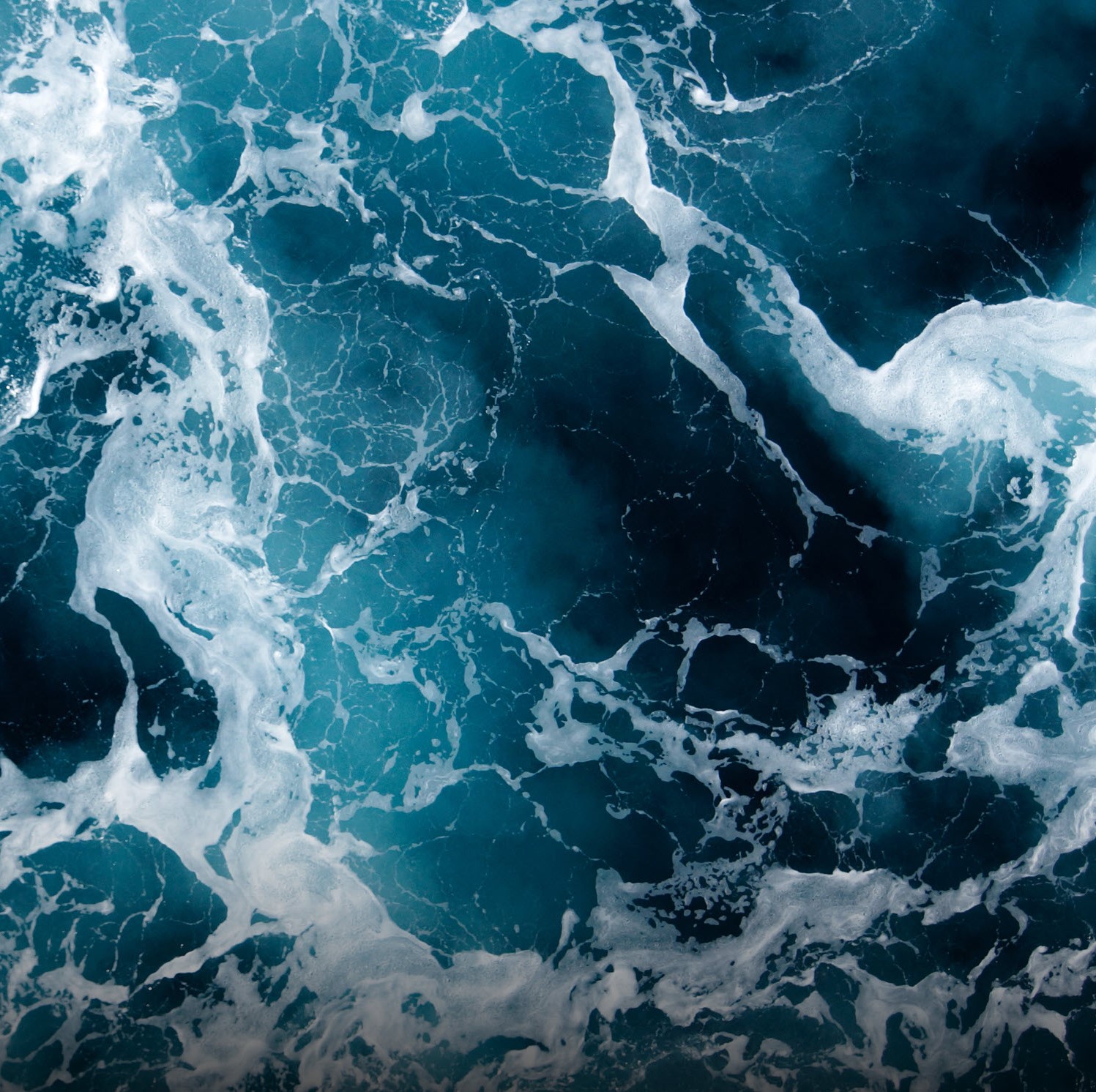
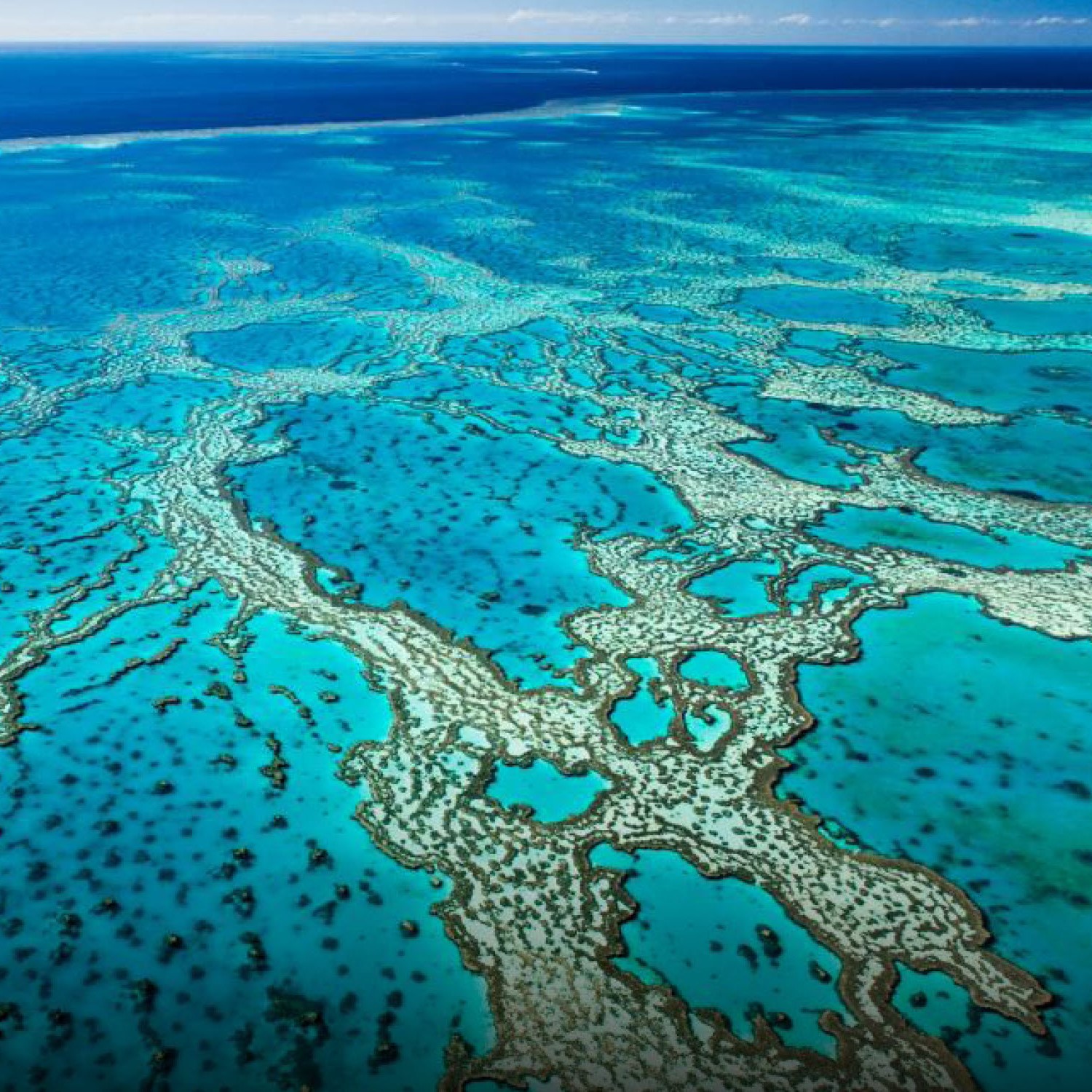